By taking advantage of reduced inaccuracies and improved productivity thanks to AI, the oil and gas industry can enjoy faster data analysis and more informed business decisions.
Beginning in the early 2000s, the term “digital transformation” became a buzzword in the oil and gas industry. Nearly every major energy company set its sights on creating a “Digital Oilfield” that would capture vast amounts of data and allow for better logistics, analytics and modeling. Other industries managed to accomplish this by utilizing a new wave of available computing power, storage and the use of artificial intelligence (AI). Why has the oil and gas industry lagged, comparatively speaking? Why can’t major oil and gas companies manage their inventory with the speed and precision of the likes of Amazon or Google? What keeps them from creating the ideal “Digital Oilfield”?
AI Challenges in an Asset Heavy Industry
First, consider the expansive scope and scale of oil and gas assets that are currently operating in the U.S. According to Ralph E. Davis Associates, there are 469,616 active oil wells, 131 operating refineries, 1320 active terminals and, in 2019, there were 224,045 miles of active oil pipelines. Given that a single oil well can produce one terabyte of data per day, the amount and depth of data generated at every step of the supply chain is immense. However, Cisco estimates that oil and gas companies currently use only about two percent of the data that they generate due to a lack of transfer capability, storage capacity and computing power.
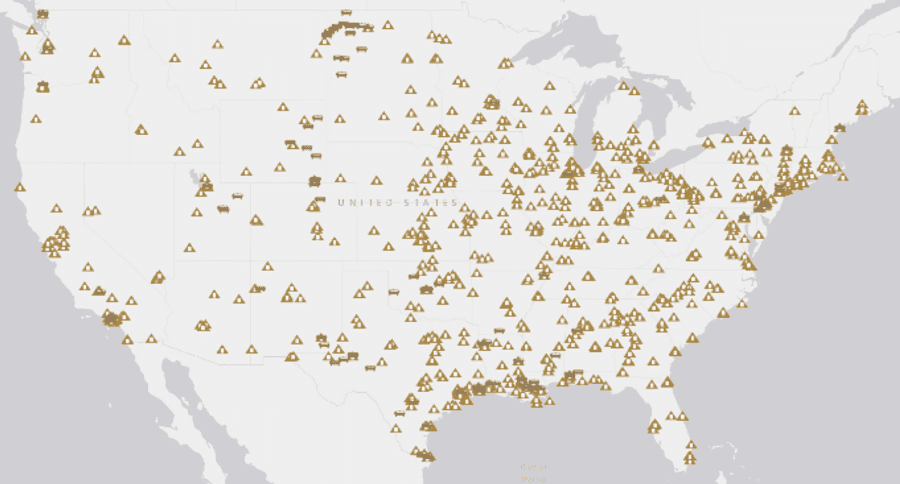
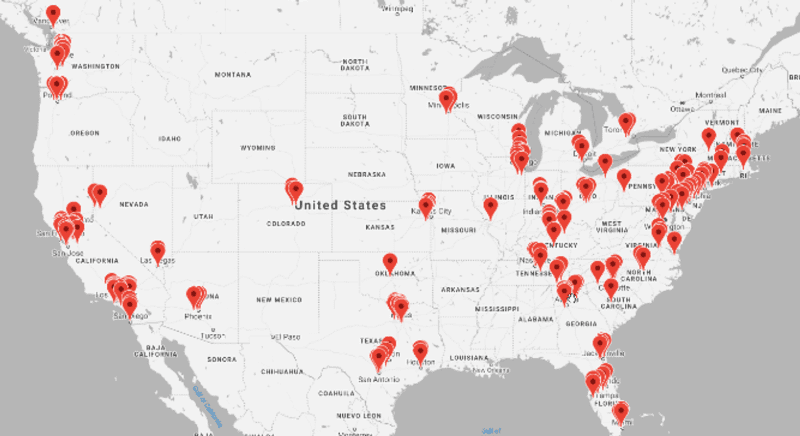
Unfortunately, it’s much more difficult to track a massive volume of crude traveling through a pipeline than it is to simply scan a radio-frequency identification (RFID) on a boxed package along its route from a warehouse to your front door. Therefore, comparing logistics involved in the oil and gas industry to a company like Amazon is a bit like comparing motorcycle repair to fixing a bicycle. Although the technology energy companies need to track, optimize and schedule logistics exists, implementing such a sophisticated system can be difficult, especially when dealing with a number of problems derived from poor data.
Where is AI Already Being Applied
Given the myriad of benefits, one would think the adoption rate of AI in the oil and gas industry would be high; however, the reality is that the degree of industry-wide acceptance is just scratching the surface. Effective implementations of AI can be seen primarily in the upstream sector. In the Permian Basin, for example, technicians historically spent much of their time driving from one remote oil well to the next checking systems and collecting data, then driving back to the central office at the end of the day and uploading it.
To reduce this inefficiency, ExxonMobil’s subsidiary XTO Energy partnered with Microsoft’s Azure AI platform to transform its daily operations by creating an AI cloud infrastructure that continuously monitors and uploads data. This not only enables technicians to focus on wells that may require more evaluation and maintenance, but it also allows for more accurate well analytics and future enhancement modeling by eliminating data silos.
How Can AI Benefit My Company?
Sometimes the hardest part of implementing an AI platform is determining where it could add value to the organization. Below are just a few examples of AI platforms that can enable oil and gas companies to operate with more agility, efficiency and accuracy than ever before.
- Exploration and production companies are using AI to analyze historical equipment data and create predictive failure analytics models, maximizing asset utilization and allowing operators to schedule maintenance and fix equipment before it fails.
- Pipelines use AI for remote monitoring of pipeline conditions, enabling a safer work environment for inspectors, and alert systems when a sensor may need to be replaced or a spill is detected.
- Refineries are able to collect, analyze and model data from over 150,000 sensors equipped in a facility and display it in the best format for a specific end-user. This allows plant operators to spend less time performing safety walks and vibration checks while improving safety in the workplace. Yield optimization machine learning software can also improve production accuracy and reduce time spent performing lab tests.
- Energy trading risk management capabilities can be further enhanced by the utilization of AI. With AI’s ability to rapidly process large datasets, coupled with its pattern recognition ability, trading surveillance processes can better isolate anomalous trading activity which may require further analysis or investigation by compliance teams.
AI platforms no longer require software companies to develop customized packages that exactly fit the needs of a user to allow for effective, fast implementation. Utilizing a low-code AI platform, users can drag and drop components to create tools at about 10 to 20 percent of the cost and time.
Overcoming AI Hurdles
Although oil and gas companies face unique challenges, there are a few things they can do to implement an effective AI platform and execute a successful digital transformation strategy. As discussed in an article written by Rob Roberts, the foundation of any AI platform implementation strategy is an effective Master Data Strategy (“MDS”). Major energy companies have operations all over the world. As groups form based on products, locations and functions, data silos filled with information can be locked away. Data silos can also lead to duplications, different classifications of the same data, and other issues stemming from a data compilation phase of an enterprise-wide software. An MDS eliminates silos and then integrates and cleanses data for AI use.
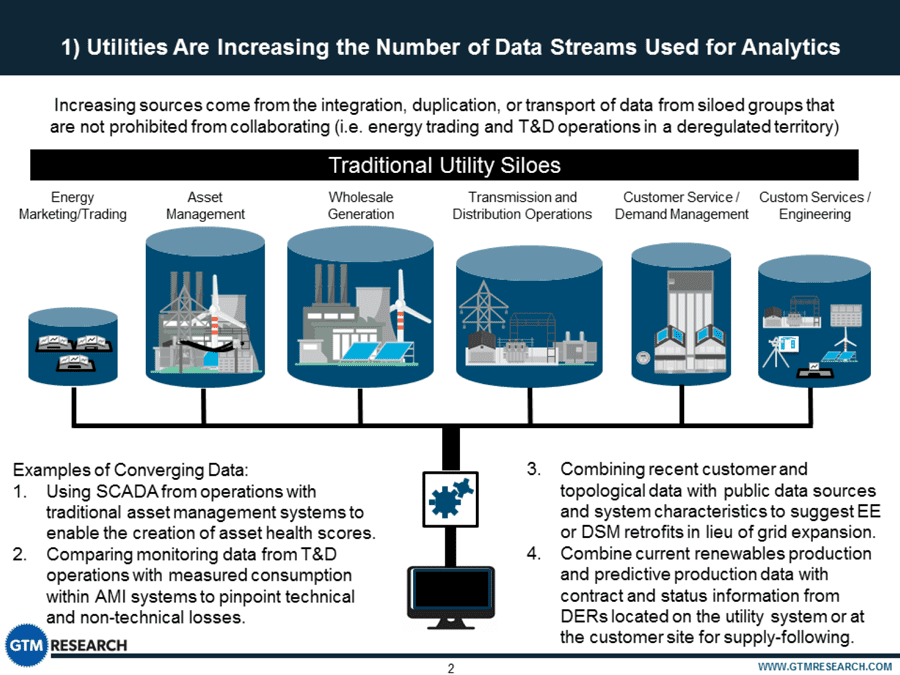
The Future Impact of AI
AI platforms require rigorous planning, building, software selection, appropriate assembly of personnel, and implementation – all of which need to be conducted in an organized manner to be effective. Rushing through any one of these phases will inevitably lead to failures and cause the entire project to lose momentum. We at Opportune stand ready to assist oil and gas companies every step of the way during their digital transformation journey.
The savings generated by AI’s analytical models have forced companies to focus on how they can use AI platforms to be more efficient, effective and get a leg up on their competition. Sometimes, all it takes is for one organization to push its limits in order to prove its effectiveness and dramatically shift the best practices of an entire industry.
The next installment of this mini-series will focus on some considerations that allow the implementation process of an AI platform to be as easy and effective as possible.
This is the first installment of a three-part series discussing AI’s potential and critical role in oil and gas, how a company can prepare for an AI platform, and important steps to executing a sound AI implementation.
Headline image courtesy of Microsoft: XTO and Microsoft Azure IoT Solution
Patrick O’Brien is an intern with Opportune LLP’s Process & Technology group. He isconcurrently pursuing an undergraduate degree in chemical engineering and an MS infinance at Texas A&M University.
Oil and gas operations are commonly found in remote locations far from company headquarters. Now, it's possible to monitor pump operations, collate and analyze seismic data, and track employees around the world from almost anywhere. Whether employees are in the office or in the field, the internet and related applications enable a greater multidirectional flow of information – and control – than ever before.